𝐩(𝐝𝐨𝐨𝐦)
No, we probably won’t all die anytime soon, but there is still a lot to be worried about.
No, I don’t think there is a 100% chance that humans will go extinct any time soon, nor a 0% chance either. 𝐩(𝐝𝐨𝐨𝐦)—I can’t figure out exactly where the term originated, and it is often used a bit tongue-in-cheek—is supposed to be the chance that we are all going to die in an extinction event.
In the limit, of course, the probability is one, and too depressing1 to talk about in polite society. When the universe goes down, all of our descendants, if we have any left, will go down with the ship.
But we blunder on. When people talk about p(doom) they are really talking about the chance that humans will go extinct soon, say in the next hundred years.
I was at a workshop in the Bay Area recently, and the “icebreaker” was, “say your name and give p(doom)”. Usually when people are talking about p(doom), they are thinking specifically about AI – could AI kill us all? What’s the odds of that? A lot of people in Silicon Valley are pretty worried that p(doom) might be something other than zero; some even seem to put the number at ten percent or higher. (Eliezer Yudkowsky seems to put it at near 100%). One survey, which was not perhaps entirely representative, put it a lot higher than that. And that was before GPT-4 which some people (not me) think somehow increases the odds of doom.
Personally, despite the name of this essay, I am not all that worried about humans going extinct anytime very soon. It’s hard for me to construct a realistic scenario of literal, full-on extinction that I take seriously. We are a pretty resourceful species, spread throughout the globe. Covid was pretty terrible, but it didn’t come remotely close to killing us all (about 1 in a 1000 humans died). Nuclear war would kill a very large number of people, and set back civilization immensely, but I doubt that literally every human in every corner of the earth would die.
But who cares what I think?
The fact that I, sitting on my couch, can’t think of a compelling scenario in which machines, directly or indirectly, on purpose or by accident, of their own volition or via the deliberate commands of a madman , could kill us all doesn’t mean it won’t happen. Poverty-of-the-imagination arguments don’t have a great track record. (Some onlookers couldn’t possibly imagine that heavier-than-air aircraft could fly, others couldn’t imagine people would be able build nuclear weapons; even in the Reagan era I didn’t imagine that a game show host could become President, etc). There are in fact already some scenarios that are faintly imaginable now (e.g. bad actors using machines to create novel toxins, in ways that go awry), and over time could be more. The reality is we just aren’t really good at imagining technology fifty or one hundred years out. Just because we can’t imagine something doesn’t mean it might now happen in 50 years. Who among us anticipated social networks with a billion users in 1990? Or any of their side effects on mental health or public discourse, and perhaps even geopolitics?
Meanwhile, p(doom) per se is not the only thing we should be worried about. Perhaps extinction is vanishingly unlikely, but what I will call 𝐩(𝐜𝐚𝐭𝐚𝐬𝐭𝐫𝐨𝐩𝐡𝐞) – the chance that an incident that kills (say) one percent or more of the population, seems a lot higher. For literal extinction, you would have to kill every human in every nook and cranny on earth; that’s hard, even if you do it on purpose. Catastrophe is a lot easier to imagine. I can think of a LOT of scenarios where that could come to pass (e.g., bad actors using AI to short the stock market could try, successfully, to shut down the power grid and the internet in the US, and the US could wrongly blame Russia, and conflict could ensure and escalate into a full-on physical war).
When Sam Altman said in May at our Senate hearing “My worst fears are that we cause significant, we, the field, the technology, the industry — cause significant harm to the world” he was not wrong. Can any of us truly say that’s simply not possible?
§
Yet a really large fraction of the AI and AI-adjacent community doesn’t really want to talk about any of this at all. Many scoff at the whole idea of discussing p(doom), and few seem to want to discuss p(catastrophe) either, when I try to bring it up. Just a couple days ago, one serious computer scientist asserted to me that “climate change is real and "AI Risk" is not”, as if we were wasting time even thinking about the problem.
One common argument is that we have no idea how to estimate it, another that most of the common scenarios proposed sound like science-fiction.
Neither counter remotely helps me sleep at night.
The problem with the latter argument is that science-fiction does sometimes come true. We didn’t have a lot of orbiting satellites when Arthur C Clarke first wrote about them, now we have thousands. Star Trek communicators came (in the form of Motorola flip phones) and have now long since been left in the dust.
The problem with the former argument—that we have no idea how to estimate p(doom)—is twofold. First, it confuses epistemology (what we can know and how we can come to know it) with probability. Just because we can’t estimate something well doesn’t mean it can’t kill us. Unfortunately, the fact that we can’t predict the course of technology with any precision even a decade out doesn’t magically immunize us from its effects, nor relieve us of a moral responsbility to try to take precautions. We can’t really estimate the probability that synthetic biology will kill us all, but we that shouldn’t stop us from taking precautions.
What’s 𝐩(𝐛𝐢𝐨𝐥𝐨𝐠𝐢𝐜𝐚𝐥 𝐜𝐚𝐭𝐚𝐬𝐭𝐫𝐨𝐩𝐡𝐞)? We probably can’t predict it with precision; there many unknowns. Any confidence interval would necessarily be broad. But does that mean we shouldn’t really worry about biological catastrophe? Or course not.
Meanwhile, we humans have a rather bad history of neglecting issues that are both serious and dark on the grounds that they rest on things we haven’t figured out. Here’s a summary of the situation with respect to climate in the 1970s:
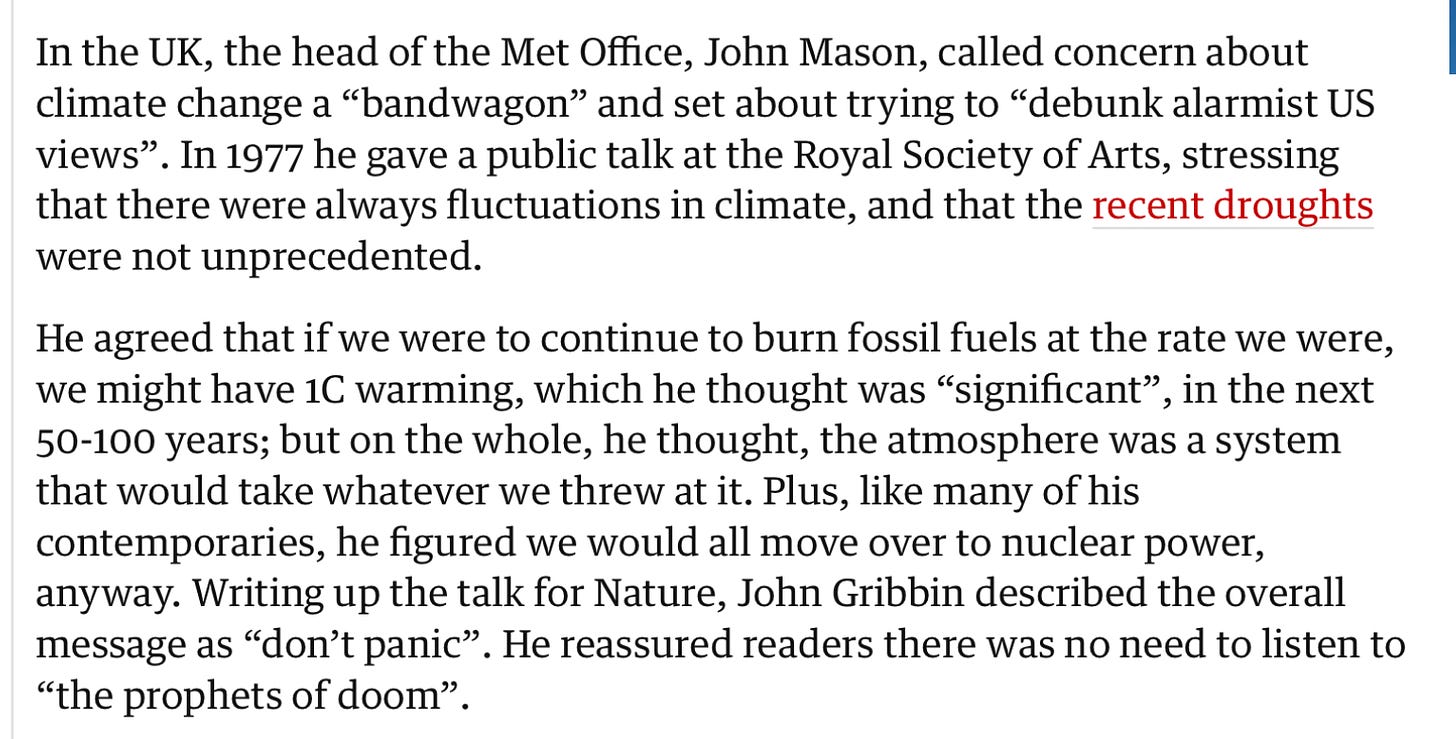
Sound familiar? The phrase 𝐩(𝐝𝐨𝐨𝐦) might be new, but the practicing of ridiculing doomers goes back at least a half century. In the case of climate change, those who did the ridicule were on the wrong side of history.
§
Another common argument is to say that there is no reason whatsoever to expect AI to control (or annihilate) humans. Yann LeCun wrote a long screed along these lines (which Steve Pinker later endorsed), saying we don’t need to worry about AI achieving dominance. Some of what he says is right, but there’s a fallacy here, too. LeCun’s premise that the smartest doesn’t always win is of course right but his argument as a whole sputters out in the end:
LeCun’s conclusion —that humans will always set the agenda—might be true, but he hasn’t given a real argument. Instead LeCun has actually assumed his conclusion—a cardinal sin in logic—as his premise. The whole thing is circular: we will be able to set the agenda therefore we will be able to set the agenda. The question is not whether we want to design AI to be like a supersmart-but-non-dominating staff member (obviously we are trying), but whether we can.
Unfortunately, LeCun gives us no hint as how to we might, and no guarantee that doing so is even possible.2
When I think about how poor his company (Meta) is even at making LLMs that don’t hallucinate, I get worried. And when I think about Meta’s track record, for emphasizing profit versus global good, I get even more worried.
If push came to shove, could we have confidence that Meta (or any other big tech company) would build maximally safe but perhaps less profitable AI rather than a maximally profitable but possibly less safe AI, given the choice?
Sometimes all you can do is laugh.
As ed tech researcher Nirmal Patel put it last night, ”Probability of AI doom depends on both technology capabilities and nature of world leaders. We have atomic bombs and one crazy leader is what it will take for doom”; eventually, we might have AI that is equally risky in the hands of a desperate, deranged leader. Should we think about how to prepare for such scenarios now? Or later?
§
None of this means of course that I suddenly think that we are close to super-smart machines. (Spoiler alert: we are not).
But if and when we get there, will they be dominating? Non-dominating? The honest answer is we haven’t a clue. Will errant dictators use them for profit or political gain? All we have is a bunch of analogies.
Which makes actually calculating p(doom) fiendishly hard. What you want is sort of like this, a calculator someone (pausai.info) is in the process of putting together on the web:
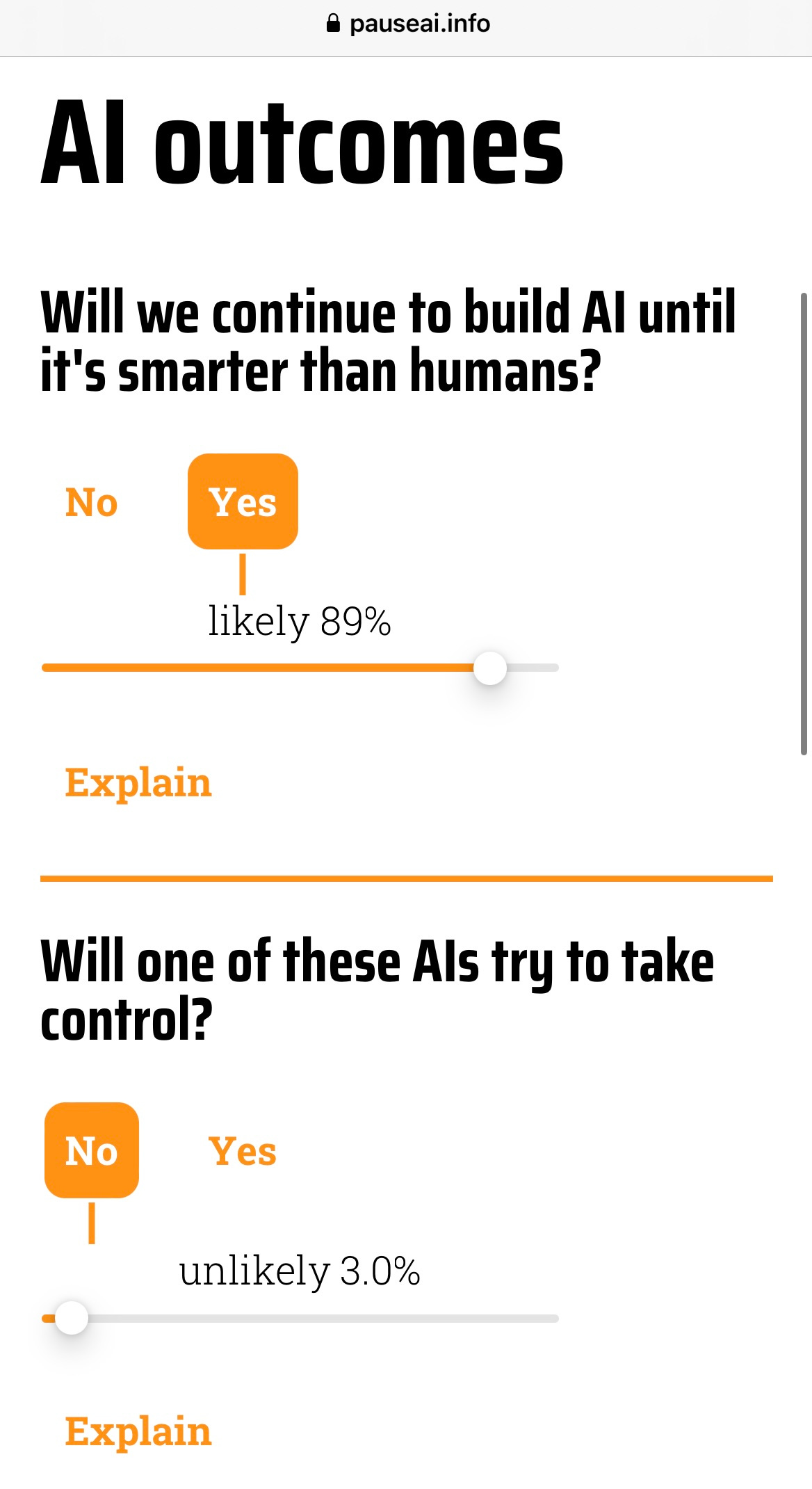
You can try it out; you set your best guesses for a bunch of sliders, and out comes your own personal p(doom) estimate, suitable for discussing at Silicon Valley cocktail parties.
§
Aryeh Englander, a graduate student at the University of Maryland, is trying to formulate these calculates this in a considerably more detailed way, writing his entire dissertation around trying to make serious, thoughtful calculations on p(doom) - and p(catastophe) as well – with proper confidence intervals, and so on. He pointed me to a 147 page analysis that already exists, and a more recent technical note from earlier this week, and hopes to produce an even deeper analysis. (As he notes the term p(doom) is itself just tongue-in-cheek shorthand, a sort of fieldwide in-joke to quickly point up some more nuanced concepts that he will treat at length.)
As Englander well knows, we will never get perfect estimates, but even imperfect estimates might help guide policy decisions as we try to balance attention to short-term and long-term risks in AI. In his words, in an email earlier this week:
We only really care about "p(doom)" or the like as it relates to specific decisions. In particular, I think the reason most people in policy discussions care about something like p(doom) is because for many people higher default p(doom) means they're willing to make larger tradeoffs to reduce that risk. For example, if your p(doom) is very low then you might not want to restrict AI progress in any way just because of some remote possibility of catastrophe (although you might want to regulate AI for other reasons!). But if your p(doom) is higher then you start being willing to make harder and harder sacrifices to avoid really grave outcomes.”
It’s therefore worth getting a rough idea of the number, even if we can’t achieve consensus on the exact number. With policy decisions at stake, the more confidence we can place in the estimate, the better. But imprecision is not an excuse for inaction.
§
I don’t self-identify as a “doomer” (unlike say Eliezer Yudkowsky who may). Unlike Yudkowsky I don’t think the probabilities are high enough—or imminent enough- to warrant bombing data centers any time soon. (Or probably ever.)
But all the ridicule I am seeing just doesn’t make sense. Some of the specific arguments Yudkowsky and Bostrom have made may well be weak, but there is no solid argument that the number is zero, or even that the risk is infinitesimal.
We waited too long on climate change; to the extent that there may be any real risk, either of outright doom or “mere” catastrophe, let’s not wait too long on AI.
§
An important clarification before I conclude, prompted by thoughtful comments on an earlier draft by Émile Torres: AI risk ≠ Superintelligence risk per se. Some of the risk from AI comes from the possibility of an uncontrollable superintelligence; a lot of it does not.
Most of the public discourse around AI risk is about whether superintelligent AI (if and when it exists) will extinguish our species - an important question that deserves answers.
But bluntly, uncontrollable superintelligence is by no means the only risk from AI, and from a policy perspective may not be the most important risk, given both the lowish (?) probability and the time horizon. I am, at least for now, much more worried about the overapplication of mediocre AI (eg what happens if we put large language models into running military strategy or into hiring decisions that affect millions of people) than the use of AI that might be so smart it could catch its own errors. And, as noted, I more worried about catastrophe than extinction per se. But I would count all of that as AI risk. I do think there is a lot of risk that AI will cause chaos. (Controllable superintelligence, by the way, has pretty serious risks, too, depending on who controls it, as Nirmal pointed above).
Within the field, there is a lot of conflict around how to think about p(doom | superintelligence) [“the probability of doom given the existence of superintelligence”, which is a special form of AI], but no matter what you feel about that p(doom | superintelligence), whether you see it as sci-fi or not, you should be worried about p(catastrophe | widespread AI adoption) [the probability of catastrophe given widespread AI adoption].
And you should care about p(catastrophe | widespread AI adoption) whatever you think about p(doom). The risks of current AI (bias, defamation, cybercrime, wholesale disinformation, etc) are already starting to be well documented and may themselves quickly escalate, and could lead to geopolitical instability even over the next few years.
And what really gets the goat of people like Timnit Gebru and Abeba Birhane, not unreasonably, is that there is a good chance that any immediate effects will disproprotionately affect the dispossessed; they are very likely right about that. Do we want, for example, a world in which rich people hire human tutors and poor people get stuck with hallucinating but cheap AI tutors that take live human teachers away from the masses? It’s a story for another day, but clearly you can have catastrophe without mass death, and we are heading in that direction.
§
One of the most intellectually honest things I have read about the whole topic is from Katja Grace, who goes through a pretty lengthy calculation on p(doom) that I won’t recount here, and then lands both on the right note of humility and the fact that we still have agency.
I’ve boldfaced the bits I am most fond of.
In conclusion: this morning when I could think more clearly 'cause I wasn't standing on a stage, I thought the overall probability of doom was 19% .. but I don't think you should listen to that very much 'cause I might change it tomorrow or something.
I think an important thing to note with many of the things in these arguments is that they could actually be changed by us doing different things. Like if we put more effort into figuring out what's going on inside AI systems, that would actually change the ‘how likely is it that we can't make AI systems do the things we want?’ parameter."
Words to live by. We aren’t going to be able calculate p(doom) to three decimal places. But let’s not give up on our efforts to ponder on the future just because it’s hard to identify it with certainty, nor become fatalists. Let’s see what we can do to maximize our chances.
Gary Marcus is the co-author of Rebooting AI, author and editor of 6 other books, founder of two companies, CEO of ca-tai.org and host of the eight-part podcast Humans vs Machines.
In the opening of Annie Hall, Alvy Singer, maybe 10 years old, talks to a psychiatrist, with his mother (Mrs Singer) in the room:
Mrs. Singer:
He's been depressed. All of a sudden, he can't do anything.Dr. Flicker:
Why are you depressed, Alvy?Mrs. Singer:
Tell Dr. Flicker. It's something he read.Dr. Flicker:
Something you read, huh?Alvy Singer:
The universe is expanding.Dr. Flicker:
The universe is expanding?Alvy Singer:
Well, the universe is everything, and if it's expanding, someday it will break apart, and that will be the end of everything.Mrs. Singer:
What is that your business? He's stopped doing his homework.Alvy Singer:
What's the point?Mrs. Singer:
What has the universe got to do with it. You're here, in Brooklyn.
At the opposite extreme, Yudkowsky claims that AGI will kill us all by default, because superintelligence will by default dominate us and harvest our atoms; this too seems like a rather large assumption that is far from proven, a possibility but not demonstrably a default,
For what it's worth, here's a slightly longer overview on my own current preferred approach to estimating "p(doom)", "p(catastrophe)", or other extremely uncertain unprecedented events. I haven't yet quite worked out how to do this all properly though - as Gary mentioned, I'm still working on this as part of my PhD research and as part of the MTAIR project (see https://www.lesswrong.com/posts/sGkRDrpphsu6Jhega/a-model-based-approach-to-ai-existential-risk). The broad strokes are more or less standard probabilistic risk assessment (PRA), but some of the details are my own take or are debated.
Step 1: Determine decision thresholds. To restate the part Gary quoted from our email conversation: We only really care about "p(doom)" or the like as it relates to specific decisions. In particular, I think the reason most people in policy discussions care about something like p(doom) is because for many people higher default p(doom) means they're willing to make larger tradeoffs to reduce that risk. For example, if your p(doom) is very low then you might not want to restrict AI progress in any way just because of some remote possibility of catastrophe (although you might want to regulate AI for other reasons!). But if your p(doom) is higher then you start being willing to make harder and harder sacrifices to avoid really grave outcomes. And if your default p(doom) is extremely high then, yes, maybe you even start considering bombing data centers.
So the first step is to decide where the cutoff points are, at least roughly - what are the thresholds for p(doom) such that our decisions will change if it's above or below those points? For example, if our decisions would be the same (i.e., the tradeoffs we'd be willing to make wouldn't change) for any p(doom) between 0.1 and 0.9, then we don't need any more fine-grained resolution on p(doom) if we've decided it's at least within that range.
How exactly to decide where the proper thresholds are, of course, is a much more difficult question. This is where things like risk tolerance estimation, decision making for non-ergodic processes, and decision making under deep uncertainty (DMDU) come in. I'm still trying to work my way through the literature on this.
Step 2: Determine plausible ranges for p(doom), or whatever probability you're trying to forecast. Use available data, models, expert judgment elicitations, etc. to get an initial range for the quantity of interest, in this case p(doom). This can be a very rough estimate at first. There are differing opinions on the best ways to do this, but my own preference is to use a combination of the following:
- Aggregate different expert judgements, quantitative models, etc. using some sort of weighted average approach. Part of my research is on how to do that weighting in a principled way, even if only on a subjective, superficial level (at least at first). Ideally we'd want to have principled ways of weighting different types of experts vs. quantitative models vs. prediction markets, presumably based on things like previous track records, potential biases, etc.
- I currently lean towards trying to specify plausible probability ranges in the form of second-order probabilities when possible (e.g., what's your estimated probability distribution for p(doom), rather than just a point estimate). Other people think it's fine to just use a point estimate or maybe a confidence interval, and still others advocate for using various types of imprecise probabilities. It's still unclear to me what all the pros and cons of different approaches are here.
- I usually advocate for epistemic modesty, at least for most policy decisions that will impact many people (like this one). Others seem to disagree with me on this, for reasons I don't quite understand, and instead they advocate for policy makers to think about the topic themselves and come to their own conclusions, even if they aren't themselves experts on the topic. (For more on this topic, see for example Why It's OK Not to Think for Yourself by Jon Matheson. For the opposite perspective, see Eliezer Yudkowsky's short book Inadequate Equilibria.)
Step 3: Decide whether it's worth doing further analysis. As above, if in Step 1 we've decided that our relevant decision thresholds are p(doom)=0.1 and p(doom)=0.9, and if Step 2 tells us that all plausible estimates for p(doom) are between those numbers, then we're done and no further analysis is required because further analysis wouldn't change our decisions in any way. Assuming it's not that simple though, we need to decide whether it's worth our time, effort, and money to do a deeper analysis of the issue. This is where Value of Information (VoI) analysis techniques can be useful.
Step 4 (assuming further analysis is warranted): Try to factor the problem. Can we identify the key sub-questions that influence the top-level question of p(doom)? Can we get estimates for those sub-questions in a way that allows us to get better resolution on the key top-level question? This is more or less what Joe Carlsmith was trying to do in his report, where he factored the problem into 6 sub-questions and tried to give estimates for those.
Once we have a decent factorization we can go looking for better data for each sub-question, or we can ask subject matter experts for their estimates of those sub-questions, or maybe we can try using prediction markets or the like.
The problem of course is that it's not always clear what's the best way to factor the problem, or how to put the sub-questions together in the right way so you get a useful overall estimate rather than something wildly off the mark, or how to make sure you didn't leave out anything really major, or how to account for "unknown unknowns", etc. Just getting to a good factorization of the problem can take a lot of time and effort and money, which is why we need Step 3.
One potential advantage of factorization is that it allows us to ask the sub-questions to different subject matter experts. For example, if we divide up the overall question of "what's your p(doom)?" into some factors that relate to machine learning and other factors that relate to economics, then we can go ask the ML experts about the ML questions and leave the economics questions for economists. (Or we can ask them both but maybe give more weight to the ML experts on the ML questions and more weight to the economists on the economics questions.) I haven't seen this done so much in practice though.
One idea I've been focusing on a lot for my research is to try to zoom in on "cruxes" between experts as a way of usefully factoring overall questions like p(doom). However, it turns out it's often very hard to figure out where experts actually disagree! One thing I really like is when experts say things like, "well if I agreed with you on A then I'd also agree with you on B," because then A is clearly a crux for that expert relative to question B. I actually really liked Gary's recent Coleman Hughes podcast episode with Scott Aaronson and Eliezer Yudkowsky, because I thought that they all did a great job on exactly this.
Step 5: Iterate. For each sub-question we can now ask whether further analysis on that question would change our overall decisions (we can use sensitivity analysis techniques for this). If we decide further analysis would be helpful and worth the time and effort, then we can factor that sub-question into sub-sub-questions, and keep iterating until it's no longer worth it to do further analysis.
The first phase of our MTAIR project (the 147 page report Gary linked to) tried to do an exhaustive factorization of p(doom) at least on a qualitative level. It was *very* complicated and it wasn't even complete by the time we decided to at least publish what we had!
A lot of what Epoch (https://epochai.org/) does can be seen as focusing in on particular sub-questions that they think are worth the extra analysis.
For more on the Probabilistic Risk Assessment approach in general, I'd especially recommend Douglas Hubbard's classic How to Measure Anything, or any of his other books on the topic.
Helpful, measured explanation. I'm amazed it is even possible to contemplate a useful approximation of p(doom) or p(catastrophe) from the multitude of (so far) predictable potential origins.